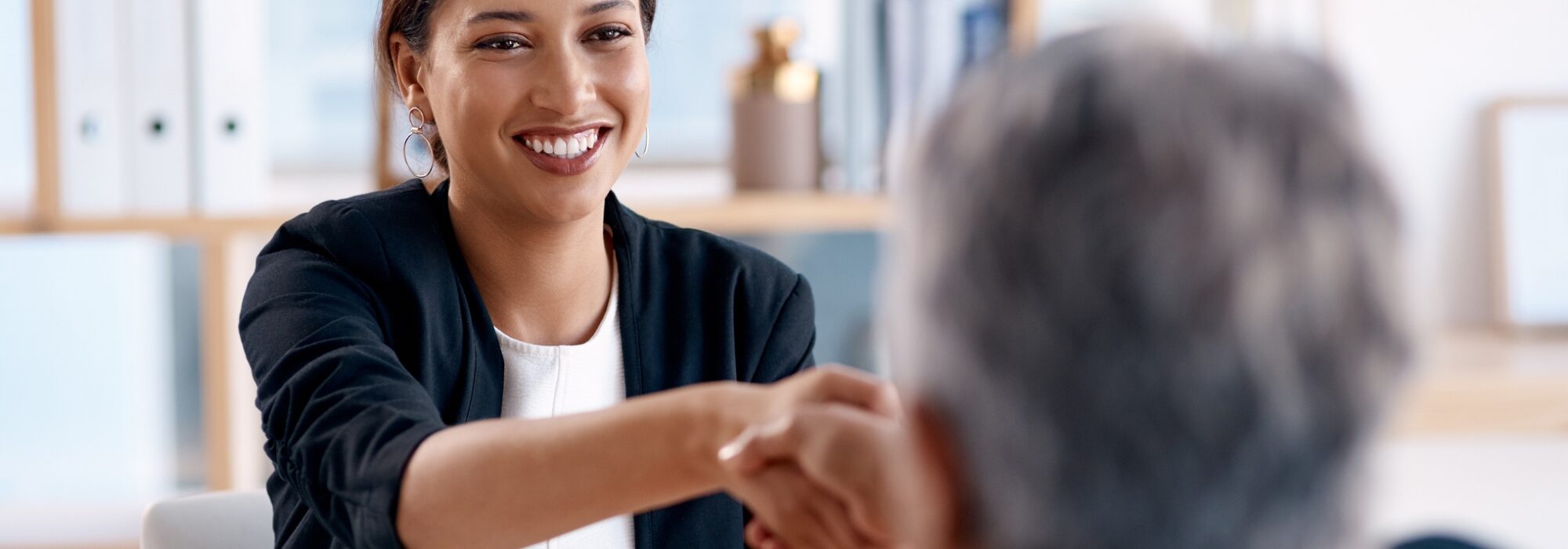
Leveraging machine learning and Big Data to enhance the customer experience
Anne Thielen, PhD, Director In-Market and Data Management at Sonova, shares three distinct ways in which we explore machine learning for predictive maintenance of hearing aids to ensure product quality and enhance the overall customer experience.
In the landscape of product quality assurance, the utilization of big data has emerged as a powerful tool for ensuring reliability and enhancing predictive maintenance strategies. As a high-quality hearing aid manufacturer, understanding the implications of big data in these domains is crucial for staying at the forefront of innovation.
The integration of Big Data in device diagnostics is based on the continuous monitoring and analysis of hearing aids as they are utilized in real-world scenarios by consumers. This is made feasible by the capability of our devices to track their own performance and health metrics, thereby providing us their diagnostic insights – all while safeguarding the privacy of our clients.
Maximizing insights with machine learning integration
By collecting health and performance metrics of hearing devices, and applying machine learning (AI) to it, usually undetected faults or issues can be identified in-real time, allowing for proactive maintenance and minimizing downtime.
In the long run, integration of machine learning enables predictive maintenance. This ensures that we can not only address potential issues promptly but also anticipate maintenance needs, ultimately enhancing the customer experience and product longevity.
Three ways Sonova is exploring Big Data analytics and applying machine learning:
1. Utilizing real-time insights
In our efforts to ensure the best quality for our customers, we have been exploring the potential of big data for reliability monitoring and predictive maintenance. Our hearing aids out there in the field continuously provide data on their performance, allowing for ongoing assessment. Examples include streaming stability, battery performance and cable breakage.
2. Proactive issue identification
By analyzing this data and employing machine learning techniques, we can identify potential issues in real-time. If technical components behave out of their specifications, like an unusual battery drop happening to a significant number of users, the information is transmitted to us within days. We can see that change in the data points right away and act on it. This proactive approach enables Sonova to address concerns promptly, minimizing disruptions for our customers and enhancing overall satisfaction.
3. Implementing predictive maintenance
Furthermore, leveraging big data allows for predictive maintenance. By analyzing patterns and trends, we can anticipate maintenance needs before they arise, ensuring our products remain in optimal condition. This predictive capability enhances efficiency and reliability. With fewer unexpected issues to address, HCPs can allocate more time to seeing additional customers, ultimately improving their practice’s productivity and patient care.
In the realm of reliability monitoring and predictive maintenance, applying machine learning to big data offers substantial benefits. It provides valuable insights that can inform decision-making and enhance the customer experience. Let’s embrace this innovative approach to ensure the continued excellence of our products and services.