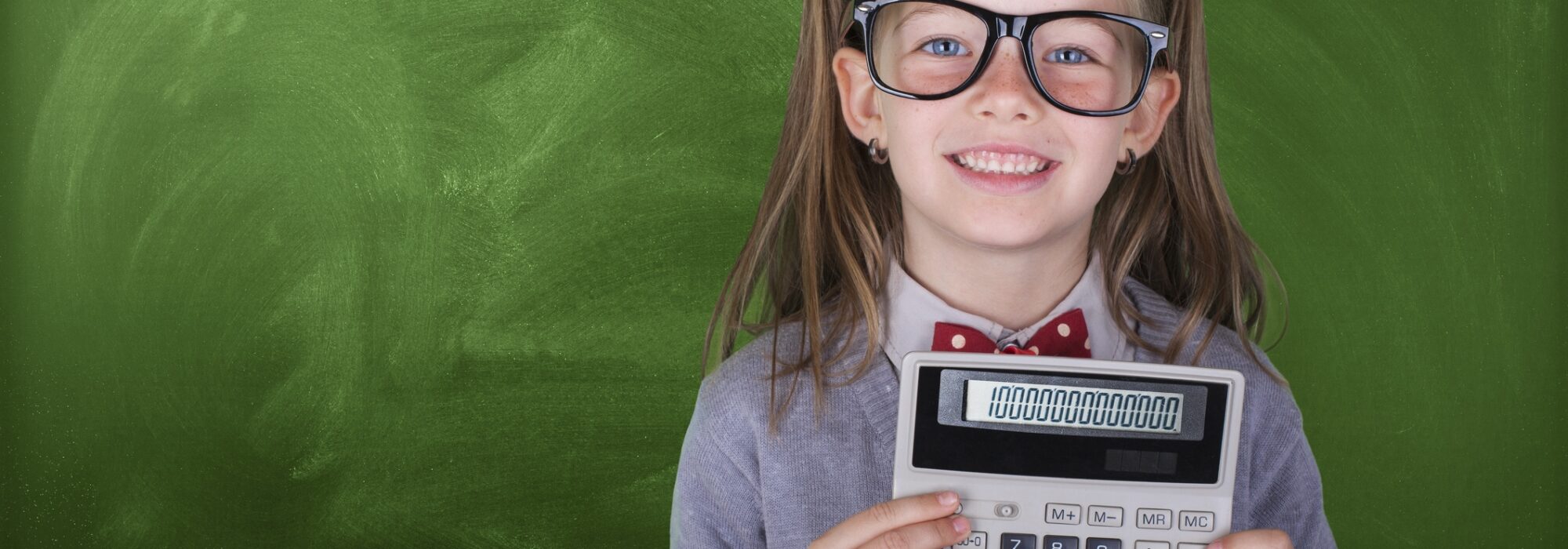
The role of statistical analyses in making sense of scientific study results
Understanding the basics of statistical analyses might tell you whether research findings are consequential or coincidental. PARC researcher Elizabeth Stewart, shares a quick tutorial and her 5th grade learnings that inspired her.
My working title for this article was, “The Time Mythbusters* Failed to Cite My Fifth Grade Science Project”
Okay, so to be fair to the producers of the long-running Discovery Channel show, I didn’t actually publish my tri-fold display board from my elementary school science fair, so they didn’t exactly steal my study idea, but they did replicate it.
Let me back up.
The idea for my 5th grade science project originated en route to a violin lesson on a drizzly day. My mom remarked that she needed to have the windshield wipers on a higher speed while we were driving than when we were sitting at a stoplight.
Did that mean that when you run through a downpour, you’re actually getting wetter than you would if you were to just walk?
More than a decade later, Mythbusters asked the same question. They even used similar methodology to answer it: in both studies, an absorbent material was weighed before and after being passed through a makeshift rain shower at different speeds.
Our results, however, were inconsistent: 11-year-old me found that the material that ‘walked’ through the rain got more saturated than the material that went faster and therefore spent less time in the rain, while the Mythbusters concluded from their data that you actually get more drenched when you cheese it through a downpour than if you were to take it slow.
So, which outcome is correct?
Though I wouldn’t blame you for taking the word of a well-funded team of experts with decades of experience over that of a literal child (though I would mention that the show revisited this myth two years later and, that time, replicated my 5th grade findings), determining which result is ‘true’ isn’t really possible because the two studies also share a major limitation: neither included any statistical analyses of the data.
Statistics are everywhere and whether you’re conscious of it or not, you engage with them every day: you check the weather forecast before heading out the door, your car tells you how fuel efficient it is via the MPG readout, your favorite streaming service shows you what content is trending.
In scientific research, statistics help to make sense of the things we observe in an experiment, determine how that information can be useful in a broader context, and most importantly, get published (kidding… sort of).
First, some basic terminology
We use descriptive statistics to summarize the characteristics of the data we have collected from our sample – that is, a subset of individuals or observations which have been selected from (and ideally, represent the characteristics of) a larger group. These include the sample mean (i.e., average), which tells us about the sample data’s central tendency, and the standard deviation, which is one way of determining how spread out the data are around the sample mean.
Inferential statistics, on the other hand, provide a systematic way to interpret the data we’ve collected and make conclusions or predictions about the population (i.e., the entire group of people or events we are interested in) that the sample represents.
At this point, I’m now understanding why most people who explain statistics do so by using an example, and I’m going to do the same (this isn’t just convention; for some reason, generalities seem inadequate when it comes to statistics).
‘The belly rules the mind’: an example about food
Let’s say – purely hypothetically – that one of your comfort foods is blueberry pancakes, and you want to know where you should go to get the bluest berry pancakes the next time you need carbs to ward off the existential dread.
So you go to your local International House of Pancakes (IHOP), order a short stack, and count the number of blueberries in each one. Then you go to the nearest Denny’s diner and do the same thing. You find that, while the number of berries isn’t exactly the same in each pancake, on average, you get 23 blueberries per IHOP pancake, and 18 per Denny’s pancake.
So if your objective is to get the most blueberry for your buck, you should always go to IHOP, right? But what if IHOP doesn’t always have extra-fruity flapjacks, they just happened to on that specific day? One way you could be more certain about who wins this particular contest is to collect more data (i.e., eat more pancakes).
The more times you repeat the measurement and get consistent results, the more confident you can be that your conclusion is true. However, there is still the possibility that your set of observations happened simply by coincidence. This is the uncertainty that researchers work to minimize by including an adequate number of observations and/or individuals in their study sample and carefully controlling the conditions of the experiment.
How the professionals analyze – a Phonak research study
In a more relevant example, Voss and colleagues wanted to know if the motion-based beamformer steering functionality available in Phonak Audéo hearing devices improves speech understanding while walking (Voss et al., 2021). To answer this question, they enrolled 20 participants of similar age and degree of hearing loss, and asked them a simple question spoken by one of the experimenters, who moved alongside (and slightly behind) the participant as they walked along an outdoor track.
Each participant completed this task twice: once with the motion-based beamformer activated, and once with it disabled. Performance in each condition was determined by the number of times the question needed to be repeated for the participant to understand it, and the accuracy of the participant’s response.
Results showed that, on average, participants were more accurate in responding to questions on the first attempt when motion-based beamformer steering was activated, compared to when this feature was disabled. The authors reported that this difference in performance between the two beamformer settings was statistically significant, as their statistical analyses of the data yielded a p value of less than 1% (i.e., p<.01).
Mind your p values
The p value expresses the estimate of the probability that the observed difference in the thing you’re measuring – your dependent variable (e.g. blueberries, raindrops, speech understanding) – occurred due to random chance and not because of an effect of your independent variable (e.g., diner chain, gait speed, beamformer setting).
The precise way this value is determined would take a good deal more explanation than is possible here, but what it tells you is how likely you would be to get the data set you got, if the truth is that your independent variable actually has zero effect on your dependent variable.
Generally, in a behavioral science like audiology, the highest probability we are willing to accept that this will happen is 5% (p<.05).
A p value of less than .05 tells us that the probability that our results occurred just by accident is quite low, so we report our findings as being statistically significant. (Of course, if that probability is actually less than 1% [p<.01], or less than .1% [p<.001], we are probably going to let you know that too.)
I should mention, however, that statistical significance isn’t the be-all and end-all of determining the success of a study (or in the case of Voss et al., the success of an intervention). This is where practical significance – or in the case of audiology, clinical significance – comes into play.
Maybe if you have hearing loss, any amount of improvement in your ability to understand your friend walking next to you is meaningful to you, regardless of whether or not the statistics found that the benefit of the motion-based beamformer was large or consistent enough to be significant.
Or maybe you think the comparative shortage of blueberries at Denny’s is no big deal, because they win on the fluffiness factor.
Or maybe you’re determined to walk through the rain no matter how soggy you get because it is just water and it’s not like you’re not being chased by a bear.
In scientific research, statistics help you make sense of study outcomes, but they don’t always tell you the whole story. On the other hand, understanding the basics of statistical analyses and how to apply and interpret them appropriately might tell you if you’re smarter than a 5th grader.
* Mythbusters is an Australian-American science entertainment television series that aired on the Discovery channel from 2003 to 2016. Using the scientific method, special effects experts test the validity of rumors, urban legends, and popular myths.
References
- Voss, S. C., Pichora-Fuller, M. K., Ishida, I., Pereira, A., Seiter, J., El Guindi, N., Kuehnel, V., & Qian, J. (2021). Evaluating the benefit of hearing aids with motion-based beamformer adaptation in a real-world setup. International Journal of Audiology, 1-13. https://doi.org/10.1080/14992027.2021.1948120